The Core Challenges in Customer Segmentation
Most businesses aim to group their customers using loyalty metrics (frequency and recency of interactions) and monetary value (e.g., Lifetime Value or LTV). However, they often face two significant challenges:
- Rigid and Arbitrary Criteria: Some companies use fixed thresholds—e.g., “frequency > 3 purchases, recency < 1 year, and LTV > $1000”—to define customer loyalty and value. While straightforward, this static approach fails to adapt to evolving market conditions and customer behavior, often rendering such definitions ineffective over time.
- Overly Complex Micro-Segmentation: On the other hand, some organizations implement exhaustive segmentation methods like RFM, which can produce an overwhelming number of segments (e.g., 64 for quartiles or 125 for quintiles). Although precise, such complexity often paralyzes marketers, leading to diluted focus and fragmented campaigns.
These extremes underline the difficulty of balancing simplicity and precision. Adaptive profiles, designed to be dynamic and scalable, provide a solution that overcomes these challenges.
The Clustered RFM Approach: A Balanced Solution
The clustered RFM model enhances traditional segmentation techniques by scoring customers on Recency, Frequency, and Monetary metrics and consolidating the results into manageable, intuitive categories. This approach combines the analytical rigor of data-driven insights with the practical simplicity marketers need for effective campaigns.
Key Steps in the Clustered Approach
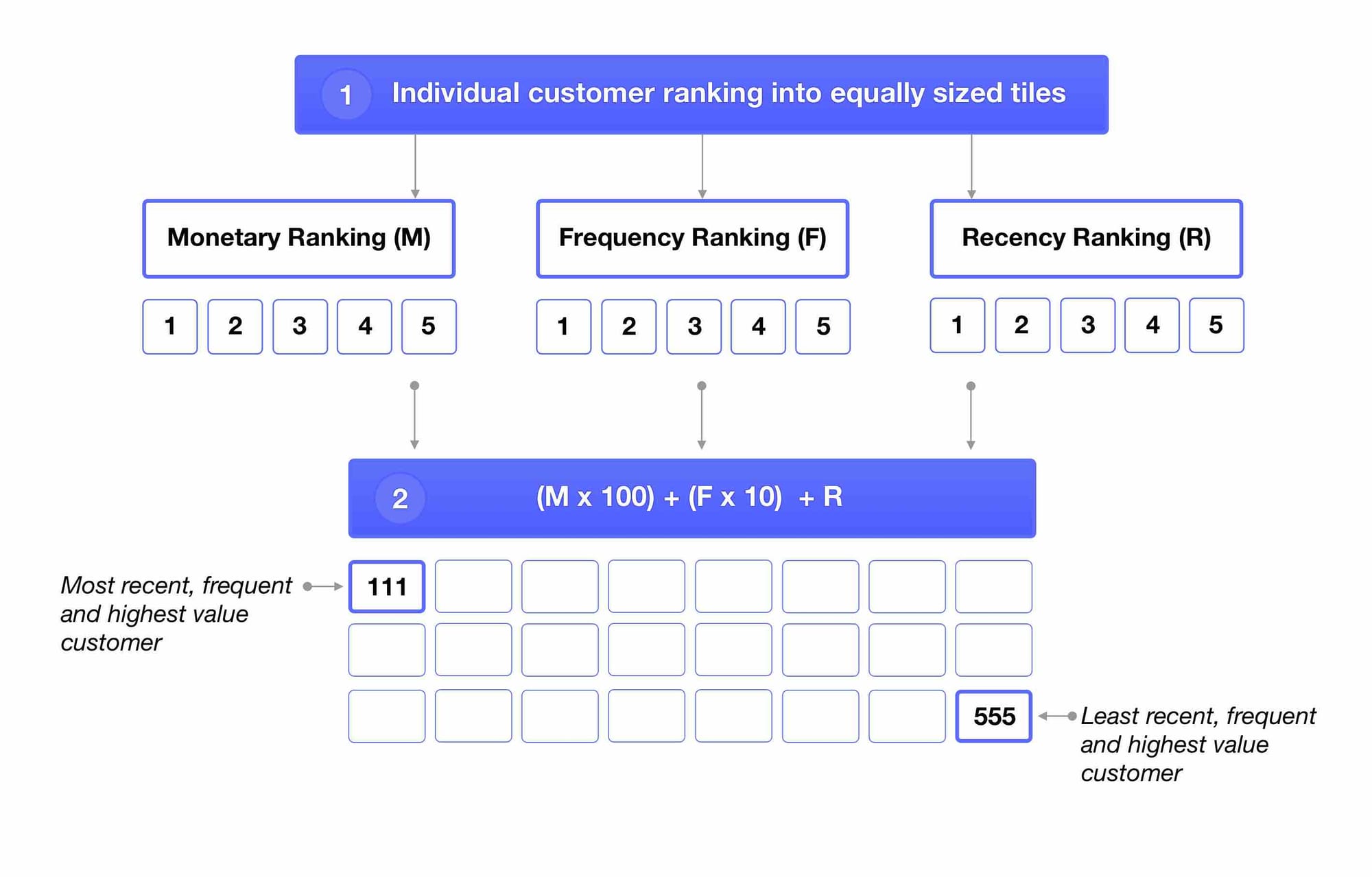
- RFM Scoring: Customers are assessed across three dimensions:
- Monetary Value (M): Ranked from 1 (lowest spenders) to 5 (highest spenders), reflecting financial contributions.
- Frequency (F): Ranked from 1 (least frequent interactions) to 5 (most frequent interactions), indicating engagement levels.
- Recency (R): Ranked from 1 (longest time since last interaction) to 5 (most recent interactions), showing their current relevance.
- Composite Scoring: These scores are aggregated using a simple formula, such as (M × 100) + (F × 10) + R. For example, a score of 111 identifies highly valuable, frequent, and recent customers, while 555 indicates low-value, infrequent, and inactive ones.
- Segment Clustering: Instead of managing a large number of micro-segments, composite scores are grouped into broader categories, such as "New," "Loyal," "Once Loyal," and "Old" customers. These actionable clusters allow marketers to focus their efforts without sacrificing strategic insights.
This approach strikes the right balance between precision and usability, making it an essential tool for modern marketers.
Visualizing Adaptive Customer Segments
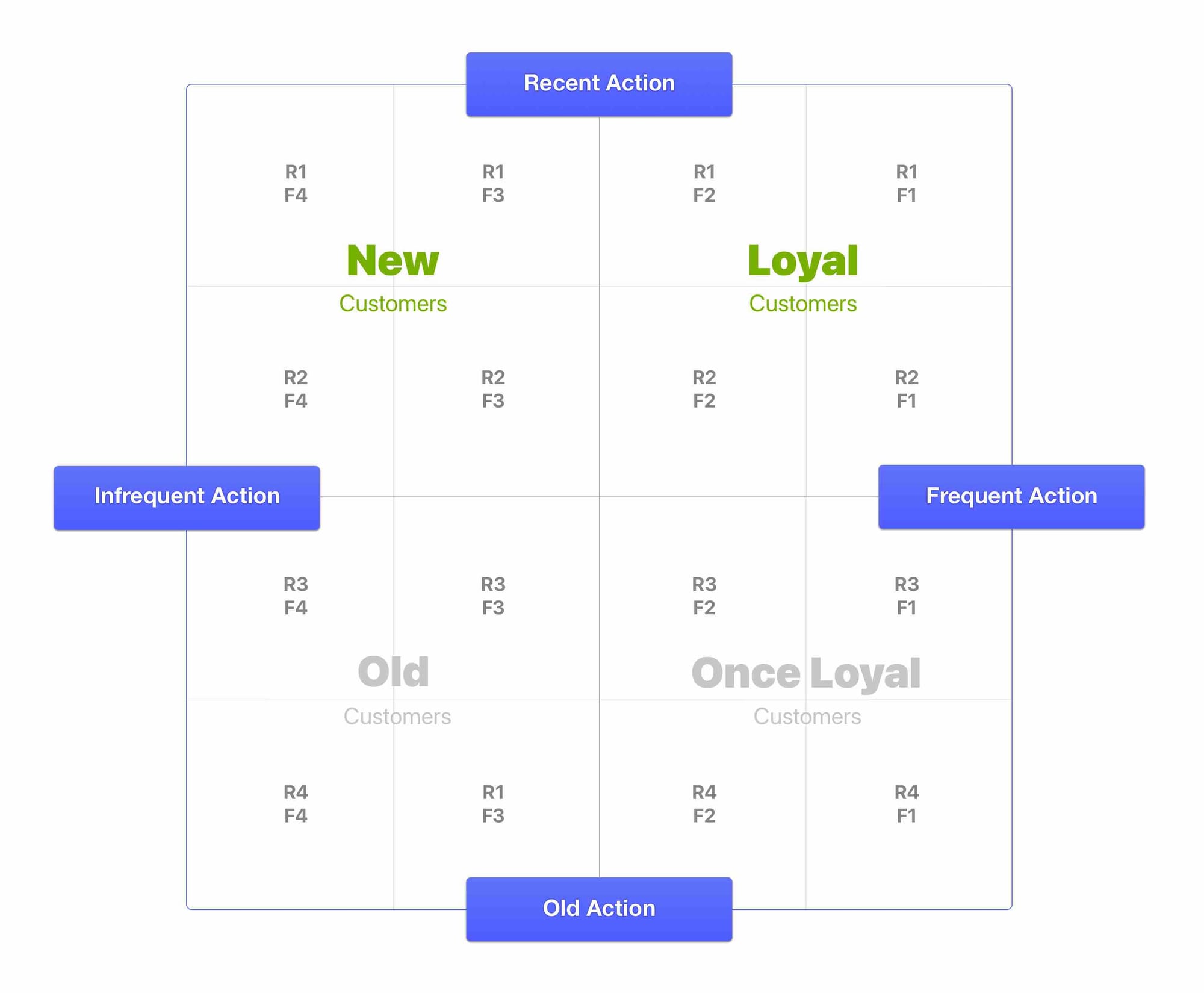
The clustered RFM model can be represented as a two-dimensional matrix:
- Recency (Vertical Axis): Reflects how recently a customer engaged with the brand.
- Frequency (Horizontal Axis): Tracks how often the customer interacts over a specific timeframe.
Using this framework, businesses can classify customers into four primary groups:
- New Customers: Customers who have engaged recently but infrequently (e.g., R1-F4). These individuals require nurturing to strengthen the relationship.
- Loyal Customers: High-frequency, recent customers (e.g., R1-F1). These customers form the foundation of the business and deserve personalized recognition and rewards.
- Once Loyal Customers: Previously frequent customers with reduced engagement (e.g., R3-F1). Re-engagement strategies are essential to recapture their interest.
- Old Customers: Low-frequency, inactive customers (e.g., R4-F4). Strong incentives may be needed to rekindle their interest.
This visualization simplifies customer behavior analysis, enabling marketers to develop targeted and impactful strategies for each segment.
Technology Solutions as an Enabler of Adaptive Profiles
Adaptive profiles thrive when paired with modern technologies that facilitate real-time data analysis and dynamic segmentation. Key technological enablers include:
- Dynamic Segmentation Tools: Platforms like Twilio Engage (formerly Segment Personas) integrate live data from warehouses and enable audience segmentation via SQL queries. These tools ensure even and consistent segmentation (e.g., by quartiles or quintiles) and adapt to changing customer behaviors.
- Computed Traits: Marketers can layer RFM insights with additional computed traits, such as brand affinities, purchase frequency, or browsing behavior. This adds depth to segmentation, facilitating more personalized and effective campaigns.
- Scalability and Adaptability: Clustered RFM models evolve alongside a growing customer base, maintaining relevance in the face of dynamic markets. These models require minimal maintenance while staying responsive to changes in customer behavior and preferences.
By leveraging these capabilities, businesses can craft adaptive profiles that are not only efficient but also tailored for impact.
Tailored Strategies for Each Segment
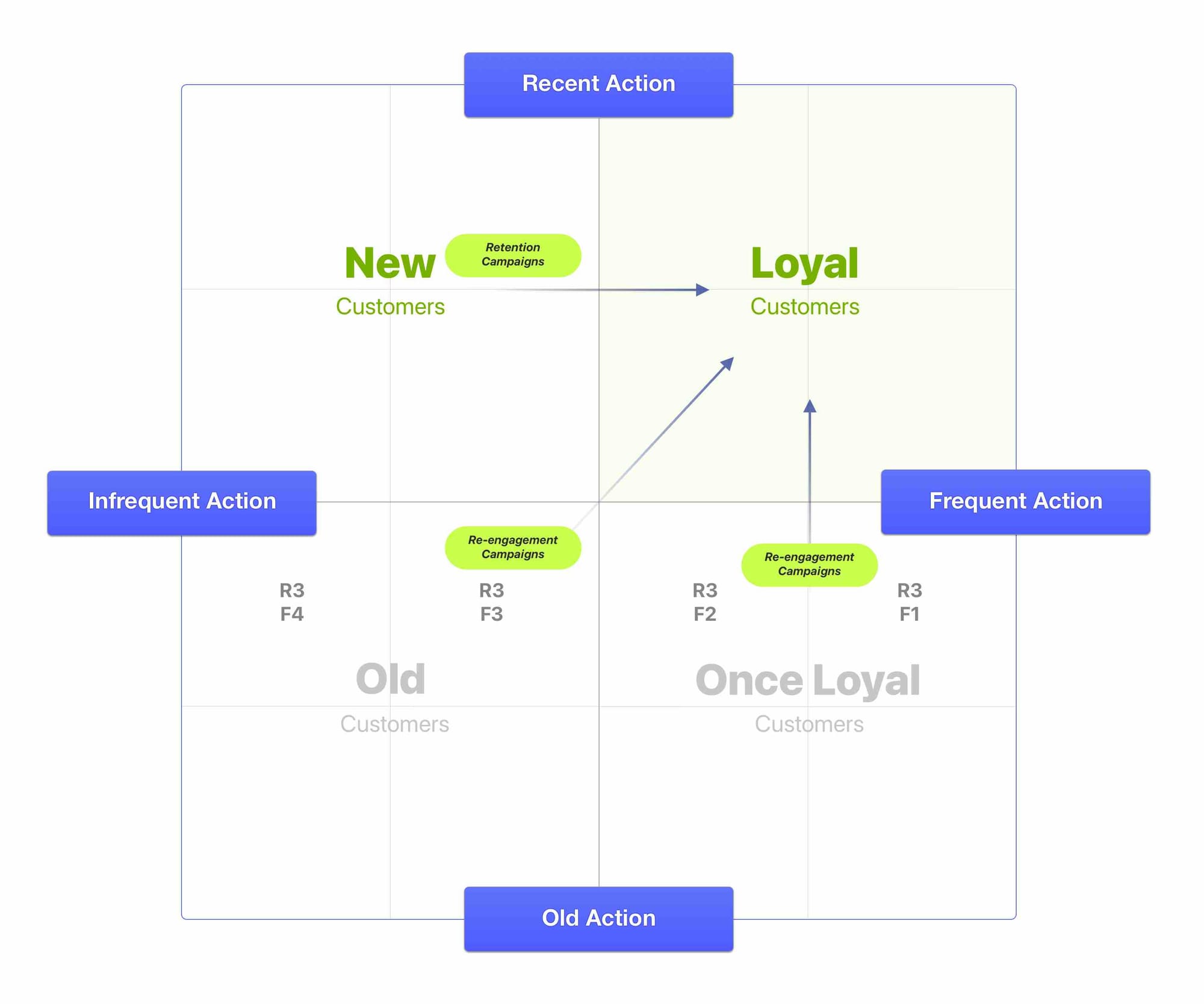
With adaptive profiles in place, businesses can design targeted strategies for each customer segment:
- New Customers: Focus on retention through onboarding programs, welcome discounts, and educational content that encourages continued interaction.
- Loyal Customers: Build loyalty with exclusive offers, personalized rewards, and invitations to VIP programs to deepen the relationship.
- Once Loyal Customers: Launch win-back campaigns using tailored promotions, personalized communications, or reminders based on past purchases.
- Old Customers: Rekindle interest with significant discounts, limited-time offers, or new product launches to encourage re-engagement.
These tailored strategies ensure campaigns resonate with customer needs and behaviors, driving both short-term conversions and long-term loyalty.
Extending the Model Across Brands
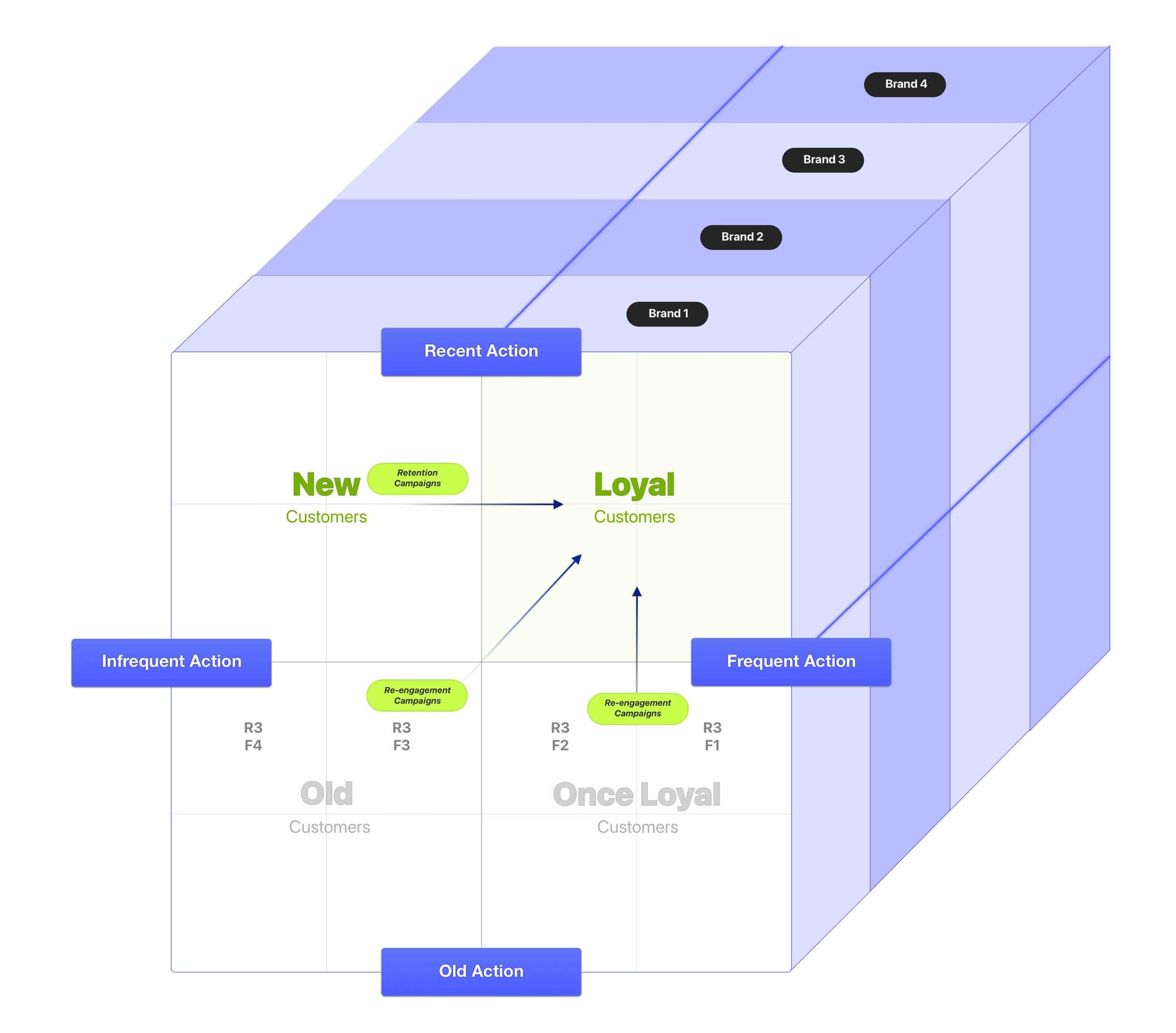
For organizations managing multiple brands or product lines, the clustered RFM approach provides a scalable framework applicable across different audiences. By maintaining a consistent segmentation strategy, businesses can:
- Compare customer behaviors across brands.
- Identify overarching trends and insights.
- Share successful campaign tactics between brands, creating synergies that boost overall performance.
This approach ensures coherence across the organization while enabling brand-specific customization for greater effectiveness.
Conclusion
Adaptive profiles, powered by clustered RFM models, offer a robust solution to the challenges of customer segmentation. By combining analytical precision with practical simplicity, they help businesses unlock actionable insights, reduce campaign complexity, and respond dynamically to customer behavior. Through tools like real-time data querying and computed traits, marketers can create segments that drive engagement, loyalty, and lifetime value.
As customer bases grow and diversify, the adaptability of this approach ensures its continued relevance. By embracing adaptive profiles, businesses can anticipate customer needs, deliver exceptional experiences, and maintain a competitive edge. For organizations looking to transform data into a catalyst for growth, adaptive profiles are the key to sustained success.